Breadcrumb
Single Volume Lung Tissue Mechanics
Local tissue expansion of the lungs is typically derived by registering computed tomography (CT) scans acquired at multiple lung volumes. However, acquiring multiple scans incurs increased radiation dose, time, and cost, and may not be possible in many cases, thus restricting the applicability of registration-based biomechanics. We propose a generative adversarial learning approaches for estimating local tissue expansion directly from a single CT scan. In a preliminary investigation, the proposed framework was trained and evaluated on 2500 subjects from the SPIROMICS cohort. Once trained, the framework can be used as a registration-free method for predicting local tissue expansion.
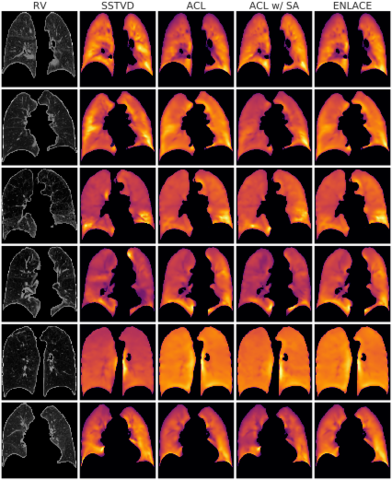